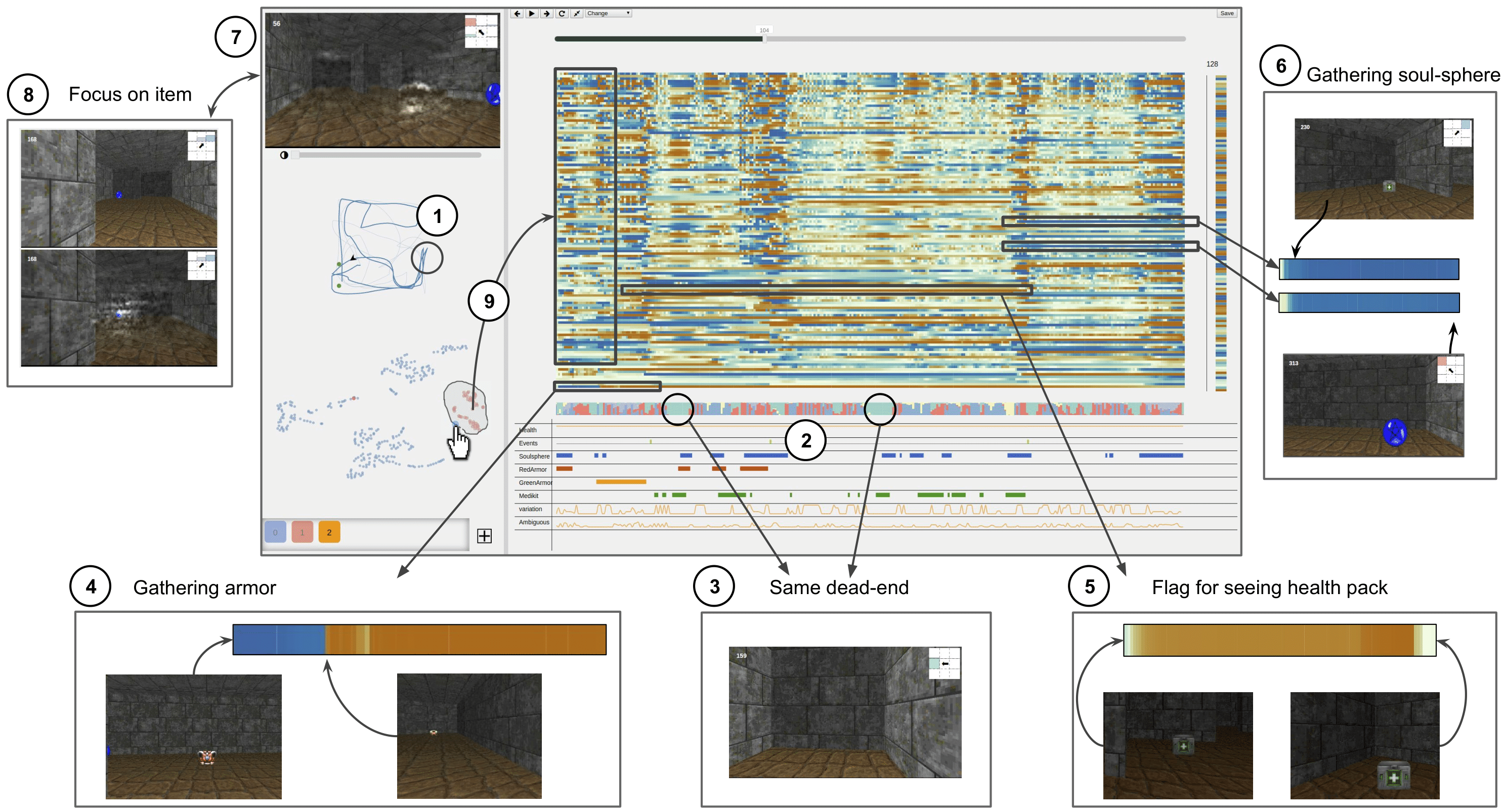
In the trajectory ① and stacked area chart of actions distribution ② we can notice two intervals during which the agent only turned right. In those intervals, the agent came twice in the same dead-end ③. In hidden states a dimension was blue when the agent sees the red armor before the green armor, and then remained orange until when saw the green armor ④. Another dimension was active as the agent first saw the HP, and remained active until it gathered it. Two hidden state elements that change as the agent gathered the health pack and then kept their values until the end of the episode ⑥. Using saliency maps ⑦, we can observe that the agent ignore the soul-sphere until it gathered the 3 firsts items ⑧. Finally, some clusters in the t-SNE projection seem to corresponds to the agent’s objectives e. g., gathering the green armor ⑨.
Abstract
We present DRLViz, a visual analytics interface to interpret the internal memory of an agent (e.g. a robot) trained using deep reinforcement learning. This memory is composed of large temporal vectors updated when the agent moves in an environment and is not trivial to understand due to the number of dimensions, dependencies to past vectors, spatial/temporal correlations, and co-correlation between dimensions. It is often referred to as a black box as only inputs (images) and outputs (actions) are intelligible for humans. Using DRLViz, experts are assisted to interpret decisions using memory reduction interactions, and to investigate the role of parts of the memory when errors have been made (e.g. wrong direction). We report on DRLViz applied in the context of video games simulators (ViZDoom) for a navigation scenario with item gathering tasks. We also report on experts evaluation using DRLViz, and applicability of DRLViz to other scenarios and navigation problems beyond simulation games, as well as its contribution to black box models interpretability and explain-ability in the field of visual analytics.

@article {10.1111:cgf.13962,
title = {{DRLViz: Understanding Decisions and Memory in Deep Reinforcement Learning}},
author = {Jaunet, Theo and Vuillemot, Romain and Wolf, Christian},
journal = {Computer Graphics Forum},
year = {2020},
publisher = {The Eurographics Association and John Wiley & Sons Ltd.},
ISSN = {1467-8659},
DOI = {10.1111/cgf.13962}
}